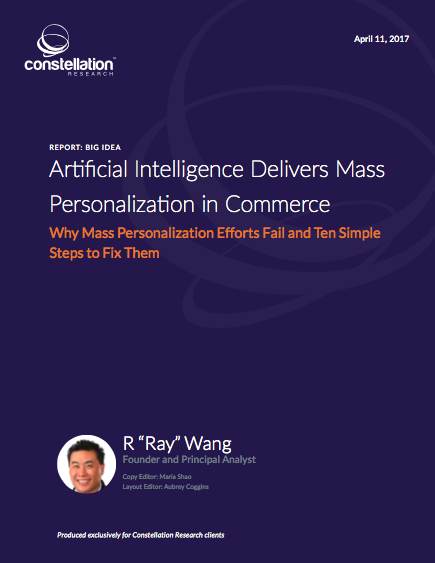
Research Summary: Artificial Intelligence Delivers Mass Personalization In Commerce
Why Mass Personalization Efforts Fail and Ten Simple Steps to Fix Them
Over the past four decades, valiant attempts at personalization have failed due to the lack of relevant and intelligent automation. Moreover, expectations of consumers and prospects have only grown. The result - an expectations gap in personalization that manifests itself in fickler consumers and greater unpredictability in revenues for brands and retailers. The inability to relevantly connect and effectively engage with consumers reflects some underlying truths:
- Stakeholders expect mass personalization. In an age of digital disruption, customers, partners, suppliers, and employees have grown accustomed to massive market choice, a plethora of pricing and policy options, and convenient delivery. The rise in expectations creates an insatiable cycle of satisfaction and disappointment that an omni-channel approach alone cannot deliver. Today, omni- channel plays only a temporary role as organizations must progress forward.
- Lack of relevance leads to lack of engagement. Contextual relevancy can be correlated to an immediate effect on the top line. Constellation estimates that lack of content relevancy often results in 83 percent lower response rates in the average marketing campaign. Conversely, personalized contextual relevancy by time of day, geo-spatial location, weather, and identity improves commerce conversions between two to three times over normal non-personalized campaigns. Context provides brands and organizations with the relevancy to earn the permission to engage with customers.
- Context provides brands and organizations with the relevancy to earn the permission to engage with customers. Manual management of personalization overwhelms most organizations. Legacy approaches are not designed for creating large-scale individualization and cannot be retrofitted. These systems classify individuals into forced-fit, binary segments. Often, individuals who belong to multiple segments and use cases are frustrated with this approach. Sadly, existing systems fail to handle the management of rules engines, policies, complex event processing, and preferences at the segment level - never mind on the individual level. Those who attempt manual personalization ultimately fail due to the complexities in managing personalization without using much technology. Moreover, sales, marketing and distribution systems must scale from hundreds of thousands to billions of customers.
- Static systems miss emerging market shifts. Technologies can no longer be static. Legacy personalization systems deceptively start out easy and end up as cumbersome anchors years later. In an era of dynamic markets, supporting technology must identify new demand signals; assess, analyze, and act on new demand signals; and apply cognitive and machine learning capabilities to adapt.
Designing AI-Driven Smart Services Starts with the Orchestration of Trust
Currently, the fashionable approach is predictive. Prediction does a great job of using past history to foretell future patterns. An intention-driven system tests for shifts in patterns by setting up hypotheses and awaiting the results. If one knows a person always gets a specific type of coffee at the same time every day, that’s predictive. An intention-driven system will test to see what type of coffee is purchased based on time of day, weather, relationships, location, and even sentiment gathered from heart rate or actions. The test comes from an offer or from studying shifts in patterns and behaviors. This self-learning and adjusting capability is powered by cognitive computing approaches. In fact, this algorithm-driven intelligence eventually will think on its own.
Digital transformation describes a shift in business models and approaches to engagement with customers, prospects, partners, employees and suppliers. AI-driven smart services provide the backbone behind these business model transformations. Consequently, crafting AI-driven smart services requires a shift in thinking to atomic-driven smart services. In fact, these new AI-driven smart services rely on five key components (see Figure 1):
Figure 1. The Secret to Designing Atomic AI-Driven Services.
Source: Constellation Research
- Digital footprints and data exhaust use AI to build anonymous and explicit profiles. Every individual, device, or network provides some information. That digital footprint or exhaust could come from facial analysis, a network IP address, or even one’s walking gait. Using AI and cognitive reckoning, systems can start to analyze patterns and correlate identity. That means that AI services will recognize and know individuals across difference contexts and take an intention-driven approach.
- Immersive experiences go beyond omni-channel. The combination of context, content, collaboration, and channels creates immersive experiences that cater to what each individual or node requires. Context starts with attributes such as identity, relationship, roles time, location, weather, sentiment. Content includes all content types from web pages, videos, product catalogs, community pages, product listings, knowledge bases, and documents. Collaboration talks about the sense and respond feedback loops. Channels are any delivery mechanism that can be accessed by a user from mobile devices, social media, kiosks, gesture, conversations as a service, augmented reality, and other AI driven UX experiences.
- Mass personalization at scale delivers intention-driven digital services. Anticipatory analytics, catalysts, and choices interact to power mass personalization at scale. Anticipatory analytics allow customers to “skate where the puck will be.” Catalysts provide offers or triggers for response. Choices allow customers to make their own decisions. Each individual or machine will have its own experience in contexts depending on identity, historical preferences, and needs at the time. From choose-your-own-adventure journeys, context-driven offers, and multi-variable testing on available choices, the AI systems offer statistically-driven choices to incite action. With no real beginning nor ending, expect these systems to work like a Choose Your Own Adventure book. Funnels fall aside as customers, partners, employees, and vendors jump in across processes, make their own decisions, and craft their own experiences on their own terms. Journey maps must account for infinite journeys and support the customer-centric points of view.
- Value exchange completes the orchestration of trust. Once an action is taken, value exchange cements the transaction. Monetary, non-monetary, and consensus are three common forms of value exchange. While monetary value exchange might be the most obvious, non-monetary value exchange (including recognition, access, and influence) often provides a compelling form of value. Meanwhile, a simple consensus or agreement can also deliver value exchange, for instance, on the veracity of a land title or the terms of a patient treatment protocol.
- Cadence and feedback complete an AI-powered learning cycle. Powered by machine learning and other AI tools, smart services consider the cadence of delivery - one-time, ad hoc, repetitive, subscription-based, and threshold-driven. Using machine learning techniques, the system studies how the smart services are delivered and applies this to future interactions.
Bottom Line: Mass Personalization At Scale Requires A Strong AI Foundation
The market need for mass personalization at scale and the technology advances in artificial intelligence (AI) enable brands and enterprises to finally deliver on the promises of digital transformation. As new algorithm-driven intelligence improves, these AI-driven smart services have the capacity to deliver immersive experiences, mass personalization, and value exchange across different modes and cadences. Further, these systems can apply machine learning to improve their capabilities in future interactions.
This report shows how AI-driven smart services deliver on the promises of mass personalization at scale, how organizations and brands can design their own AI-driven smart services, and highlight 10 recommendations to accelerate personalization success.
Click here to purchase the report and get the 10 recommendations to accelerate personalization success
Your POV.
Are your commerce systems old and creaky? Ready to modernize commerce but don't know how? Do you have a digital transformation strategy? Looking to apply matrix commerce? Add your comments to the blog or reach me via email: R (at) ConstellationR (dot) com or R (at) SoftwareInsider (dot) org.
- Developing your digital business strategy
- Connecting with other pioneers
- Sharing best practices
- Vendor selection
- Implementation partner selection
- Providing contract negotiations and software licensing support
- Demystifying software licensing
Reprints can be purchased through Constellation Research, Inc. To request official reprints in PDF format, please contact Sales .
Disclosure
Although we work closely with many mega software vendors, we want you to trust us. For the full disclosure policy,stay tuned for the full client list on the Constellation Research website. * Not responsible for any factual errors or omissions. However, happy to correct any errors upon email receipt.
Copyright © 2001 – 2017 R Wang and Insider Associates, LLC All rights reserved.
Contact the Sales team to purchase this report on a a la carte basis or join the Constellation Customer Experience